Outstanding Tips About Why Do We Use Time Series How To Plot A Standard Curve In Excel
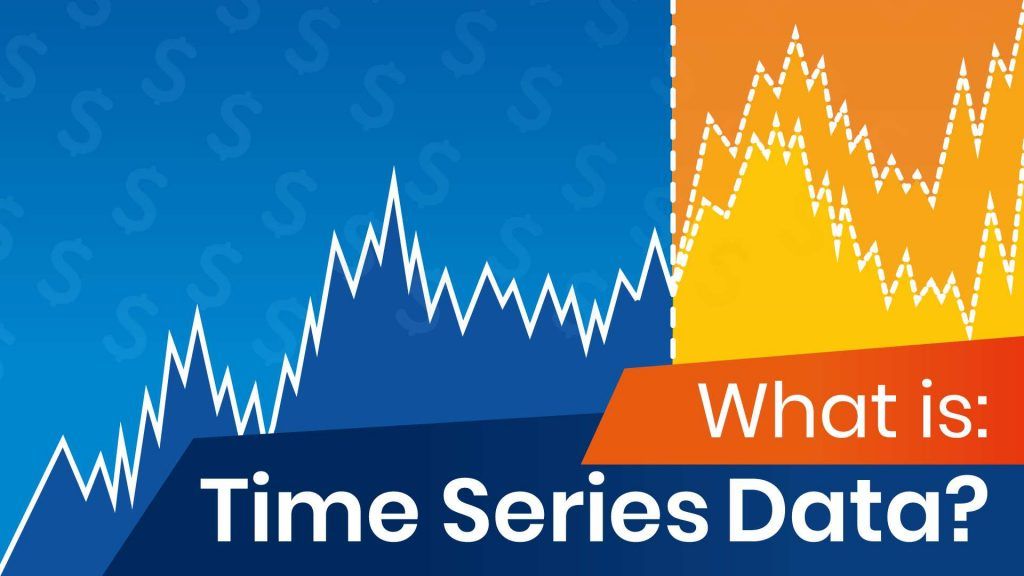
A time series with lag (k=1) is a version of the original time series that is 1 period behind in time, i.e.
Why do we use time series. Most commonly, a time series is a sequence taken at successive equally spaced points in time. Why do we need time series? In the general case, the sample mean of a stochastic process $\frac{1}{t} \sum_{t=1}^t y_t$ may not converge to anything at all!
A time series is a series of data points indexed in time order. Most people consider stock returns as more or less iid, thats why we use them. The scientific literature mostly focuses on deep learning models.
In mathematics, a time series is a series of data points indexed (or listed or graphed) in time order. Those prices were recorded daily. If you can see exactly how the price of a security has changed over time, for example, you can make a more educated guess about what might happen to the price over the same interval in the future.
So time series analysis is used to understand the determining factors and structure behind the observed data, choose a model to forecast, thereby leading to better decision making. Why use sin and cos (which map to a circle)? Hence, the strategy should always.
Stationarity is an important concept in the field of time series analysis with tremendous influence on how the data is perceived and predicted. To answer why we need time series, we need to know the vast area where they are implemented. For example, if we are interested in seasonal effects, we still have the seasonal variation we have removed, isolated from other components.
They are mostly plotted using line graphs or line charts. However, there are other aspects that come into play when dealing with time series. It offers a smooth, slippery glide and is easy to dispense from its glass pump bottle, which looks great on a nightstand.
Scientific literature had a small effect on applied time series forecasting. Use a scanner and image capture to import photos that were taken with a film camera. The key point is that when removing trend, seasonality or noise, we don't just delete information.
I have a dataset collected using an accelerometer. In this tutorial, you will discover how you can apply normalization and standardization rescaling to your time series data in python. In a time series, time is often the independent variable and the goal is usually to make a forecast for the future.
Is the target variable autocorrelated? What is the value that this phenomenon had at. It is the most expensive of our.
After completing this tutorial, you will know: We see a gap between the scientific literature and applied ml forecasting for time series. Matthew urwin | aug 01, 2023.